Fajardo Portillo, Jose Oscar
Datu pertsonalak
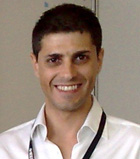
Helbidea: Alda. de Urquijo s/n. C.P.: 48013. Bilbao
Email: joseoscar.fajardo@ehu.es
Teléfono: +34 94 601 7361
Titulazio akademikoa
Unibertsitateko Titulazioa
Titulua: Telekomunikazio Ingeniaritza
Areagotzea: Telematika
Zentroa: Euskal Herriko Unibertsitatea
Lorpen data: 2003
Doktoregoa
Egitarauaren titulua: Tecnologías de la información y comunicaciones en Redes Móviles
DEA lorpen data: 2005
Título de la tesis: Adaptación de los mecanismos de provisión de calidad de servicio a las preferencias de los usuarios (Adaptation of QoS provisioning mechanisms to user requirements)
Lorpen data: (Amaiturik gabe)