Dialogue systems are a crucial component when robots have to interact with humans in natural language. In order to improve these interactions over time, the system needs to be able to learn from its experience, its mistakes and the user's feedback. This process –fittingly known as lifelong learning– is the focus of LIHLITH, a European project funded by the CHIST-ERA programme and led by the UPV/EHU's IXA and RSAIT groups.
-
In memoriam: Arturo Muga
-
Violeta Pérez Manzano: «Nire ahotsa ijito bakar batengana iristen bada eta horrek inspiratzen badu, helburua bete dut»
-
Azukrea eta edulkoratzaileak. Zer jakin behar dut?
-
Athletic zuri ta gorria, zu zara nagusia, baina zertan? Gizonezko futbol profesionalaren gaitasun (im)mobilizatzaileari buruzko hausnarketa soziologikoa
-
Iñigo Argomaniz sustatzaileak eta Eli Arabaolaza ikertzaileak irabazi dute XXIII. Donostiako Orfeoia-UPV/EHU Saria
Improving communication skills of robots through Lifelong Learning
The IXA and RSAIT groups at the UPV/EHU-University of the Basque Country is leading the LIHLITH European project, which aims to improve the self-learning capabilities of robots
- Research
First publication date: 11/07/2018
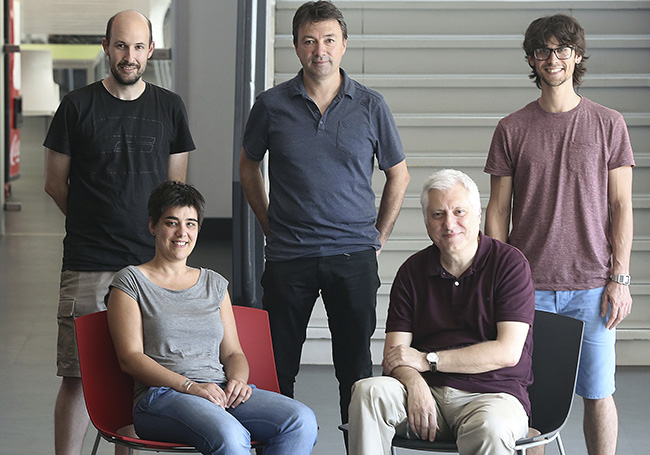
Artificial Intelligence is a field that is progressing rapidly in many areas, including dialogue with machines and robots. Examples include speaking to a gadget to request that simple tasks be performed, such as turning on the radio or asking for the weather forecast, but also more complex settings in which the machine calls a restaurant to make a reservation, or a robot assists customers in a shop.
The European LIHLITH (Learning to Interact with Humans by Lifelong Interaction with Humans) project “focuses on human-machine dialogue. It aims to improve the self-learning capabilities of artificial intelligence”, explained Eneko Agirre, researcher at the UPV/EHU. More specifically, LIHLITH will devise dialogue systems which learn to improve themselves based on their interactions with humans. It is a three-year, high risk/high impact project funded by CHIST-ERA and led by the UPV/EHU’s IXA and RSAIT research groups, which embarked on the work in January 2018. Participating partners are researchers from the University of the Basque Country (UPV/EHU),the Computer Science Laboratory for Mechanics and Engineering Sciences (LIMSI), The National Distance Education University in Spain (UNED), the Zurich University of Applied Sciences (ZHAW), and Synapse Développement in France.
Continuous improvement of dialogue systems
A chatbot or talkbot is a computer program or piece of artificial intelligence, which conducts a conversation via auditory or textual methods. Current industrial chatbots are based on rules which need to be hand-crafted carefully for each domain of application. Alternatively, systems based on machine learning use manually annotated data from the domain to train the dialogue system. In both cases, producing rules or training data for each dialogue domain is very time-consuming, and limits the quality and widespread use of chatbots. In addition, companies need to monitor the performance of the dialogue system after it has been deployed, and re-engineer it to respond to user needs. Throughout the project, “LIHLITH will be exploring the paradigm of life-long learning in human-machine dialogue systems with the aim of improving their quality, lowering the cost of maintenance, and reducing the effort involved in deployment in new domains”, said Agirre, the project’s lead researcher.
Standard dialogue systems use natural language understanding to process user input, dialogue management to access domain knowledge and decide on the next utterance, and natural language generation to produce the next utterance in the system. The main goal of life-long learning systems is to continue to learn after being deployed. In the case of LIHLITH, “the dialogue system will be developed as usual, but it will include the means to continue to improve its capabilities based on its interaction with users”, explained Agirre. “The key idea is that dialogues will be designed to get feedback from users, while the system will be designed to learn from this continuous feedback. This will allow the system to keep improving during its lifetime by quickly adapting to domain shifts that occur after deployment.”
LIHLITH will focus on “goal-driven question-answering dialogues, where the user has an information need and the system will try to satisfy this need as it chats with the user”, he said. So the project has been organised into three research areas: lifelong learning for dialogue; lifelong learning for knowledge induction and question answering; and evaluation of dialogue improvement. “All modules will be designed to learn from the available feedback using deep learning techniques. The lifelong learning module is the key innovation of LIHLITH, and will improve all modules as the system interacts with users by updating the domain knowledge accordingly”, said. The project will explore the autonomous reconfiguration of dialogue strategies and proactive capabilities to ask the user for new knowledge and for performance feedback.
To carry out this research, LIHLITH combines machine learning, knowledge representation and linguistic expertise. The project will build on recent advances in a number of research disciplines, including natural language processing, deep learning, knowledge induction, reinforcement learning and dialogue evaluation, to explore their applicability to lifelong learning.