12-01-2023;10:30 Defensa de Tesis Doctoral Onintze Zaballa Larumbe
Fecha de primera publicación: 29/12/2023
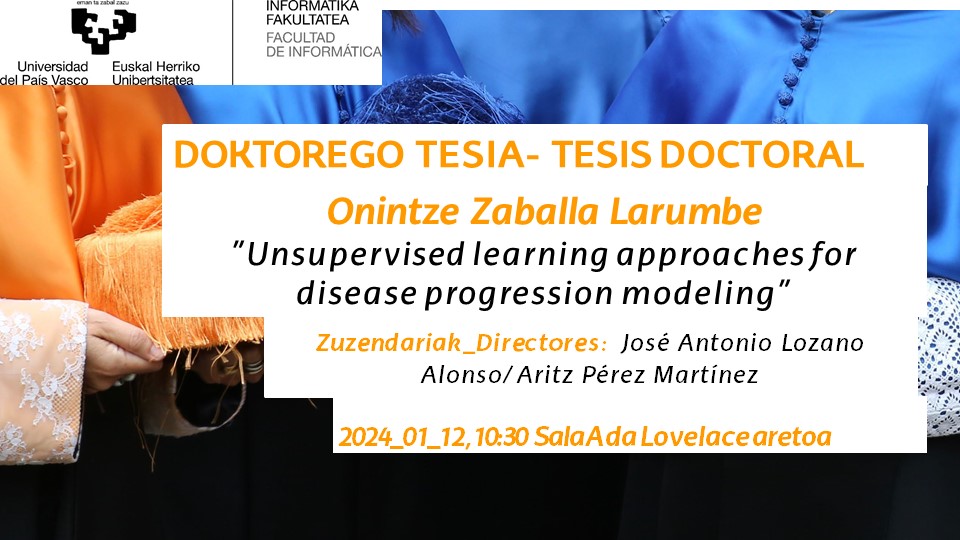
Onintze Zaballa Larumbe: ”Unsupervised learning approaches for disease progression modeling”.
Zuzendariak_Directores: : José Antonio Lozano Alonso/ Aritz Pérez Martínez
2024_01_12 , 10: 30 Sala Ada Lovelace aretoa.
Abstract:
"Electronic Health Records (EHRs), which store extensive patient and treatment data, provide an opportunity for machine learning models to capture disease progression patterns over time. Each medical record in these repositories is composed by a set of clinical variables, including a medical action, a diagnosis, and a timestamp. The medical action describes the trajectory of a patient in the healthcare system and the diagnosis associates each medical event with a specific disease. Therefore, a patient's treatment trajectory is characterized by a chronological sequence of medical records.
The primary objective of this dissertation is to develop methodologies that provide an understanding of patients' treatment progression through meaningful pattern recognition in EHRs. Generative models are powerful approaches for this purpose, as they enable the learning of the underlying data distribution, and offer an interpretable representation of disease dynamics from data. These models have additional benefits, including pattern identification, data augmentation, anomaly detection, and uncertainty estimation in predictions, among others.
In contrast to generative approaches, most existing deep learning models in healthcare focus on accurately predicting future events rather than comprehensively modeling disease progression. Understanding disease progression remains challenging for these methods due to various factors, including limited data availability, data quality problems like missing diagnosis data, and the need for interpretable results in healthcare settings. Generative models provide more interpretable patterns of disease dynamics, require less quantity of data and work properly even in the presence of missing data. Although previous generative models have advantages over deep learning models, they often make simplified assumptions for capturing the evolution of diseases. Further research is required to appropriately model key medical aspects such as the sequential occurrence and relationship of consecutive medical events, the irregular time intervals between records, and the coexistence of multiple diseases when diagnoses are missing.
This dissertation presents unsupervised methodologies to provide interpretable understanding of the progression of disease trajectories. To this end, we develop methods based on different sequence classification techniques. On the one hand, we propose a methodology based on partitional clustering for identifying disease treatment subtypes from EHRs with missing diagnosis information. Specifically, the methodology is based on the K-medoids approach with an adaptation of the edit distance, which enables to determine a representative for each subtype of treatments. On the other hand, we propose various probabilisitic generative models for sequences of medical events to analyze different scenarios in disease dynamics. The models include latent variables to capture treatment progression, temporal irregularity, and comorbidities in medical data. We introduce efficient methods for learning these models, combining the Expectation-Maximization algorithm and dynamic programming.
The effectiveness of the methodological proposals is evaluated using a real-world dataset from Osakidetza, the public healthcare system in the Basque Country, Spain. Each patient in these EHRs is represented by a sequence of medical services over time, with only 19% of these medical events having an associated diagnosis value. We include practical applications involving breast cancer patients, demonstrating the relevance and potential impact of the models. In summary, this dissertation presents methodologies that offer valuable insights into disease dynamics while addressing the unique challenges presented in EHRs".