29-02-2024; 10: 30 Defensa tesis doctoral de Aitor Ormazabal Oregi
Fecha de primera publicación: 20/02/2024
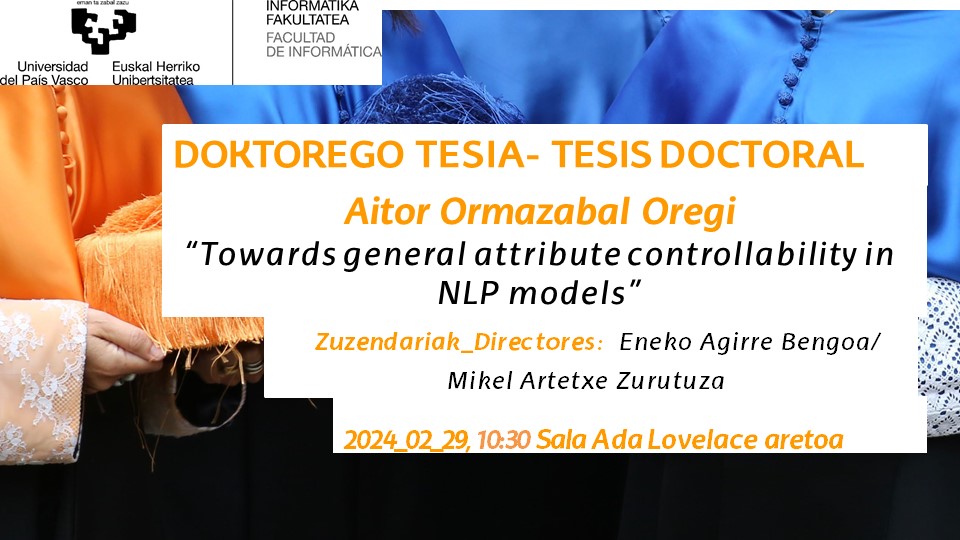
Aitor Ormazabal Oregi : “Towards general attribute controllability in NLP models”
Zuzendariak / Directores: Eneko Agirre Bengoa/ Mikel Artetxe Zurutuza.
2024-02-29, 10: 30: Sala Ada Lovelace aretoa.
Abstract:
"Advances in deep learning methodology and computing infrastructure have yielded impressive results in the field of Natural Language Processing (NLP) in recent years. However, the core paradigm followed by deep learning methods has not changed much in the past decade. Deep learning models derive their behavior entirely from their training data and learning objective, and often do not offer any mechanisms to control or steer their outputs. Thus, if one wants to control a certain aspect of the model's output, one needs to gather training data that explicitly demonstrates the desired attribute, which is not always feasible or practical.
The goal of this thesis is to address this issue by designing methods to control diverse attributes of output of NLP, beyond the existing paradigm of simply gathering more training data and re-training the model.
In the first section of the thesis we focus on unsupervised methods that allow for controllability when training data that exemplifies the desired attribute is not available. We develop three methods for different model architectures, in accordance with the evolution of the field during the development of this thesis.
First, we propose a method to control the alignment of static word embeddings during training without any bilingual supervision, and apply it to train state-of-the-art ---at the time of publication--- unsupervised bilingual word embeddings.
Second, we leverage the information bottleneck technique, together with an adversarial training setup, to control the information content in the encoded representation of a sequence-to-sequence model, and apply it to develop a paraphrase system from bilingual corpora. We prove mathematically that our method alleviates issues inherent to the popular round-trip translation baseline for paraphrasing, while offering a natural way to control the tradeoff between diversity and fidelity in the paraphrases.
Third, we explore the use of control codes to train a meter- and rhyme-controllable language model, and develop PoeLM, an unsupervised poetry generation model for Basque and Spanish. We show for the first time that control codes can be used for the control of fine-grained and strict attributes such as meter and rhyme patterns, and evaluate our method through both automatic metrics and human evaluation. We find that human evaluators often rate equally or prefer short poems generated by PoeLM to those written by layman human volunteers.
Having developed several unsupervised methods for different architectures and attributes, the second part of this thesis focuses on a general method for arbitrary adaptation of language models. Particularly, we focus on the scenario where one wants to adapt a language model when access to the internals of the model is not possible. This scenario has become particularly relevant in recent years, where, due to both the extreme scale of modern language models and the proliferation of black-box models hidden behind APIs, one often cannot simply fine-tune the model's weights for adaptation. To this end, we present CombLM, a method for black-box language model adaptation, that first trains a fine-tuned small "expert" model on the target task or domain, and then combines it with the black-box model at the probability level through a learned combination, to obtain an adapted model. Our approach allows us to leverage the deep knowledge of existing large models, while retaining the flexibility to adapt them to new domains and tasks. We show the effectiveness of our approach for adaptation to several domains and one downstream machine translation task."