2023-09-29; 10:30 DOKTOREGO TESI BATEN DEFENTSA ABDULLAH JAMAL BARADAAJI
Fecha de primera publicación: 19/09/2023
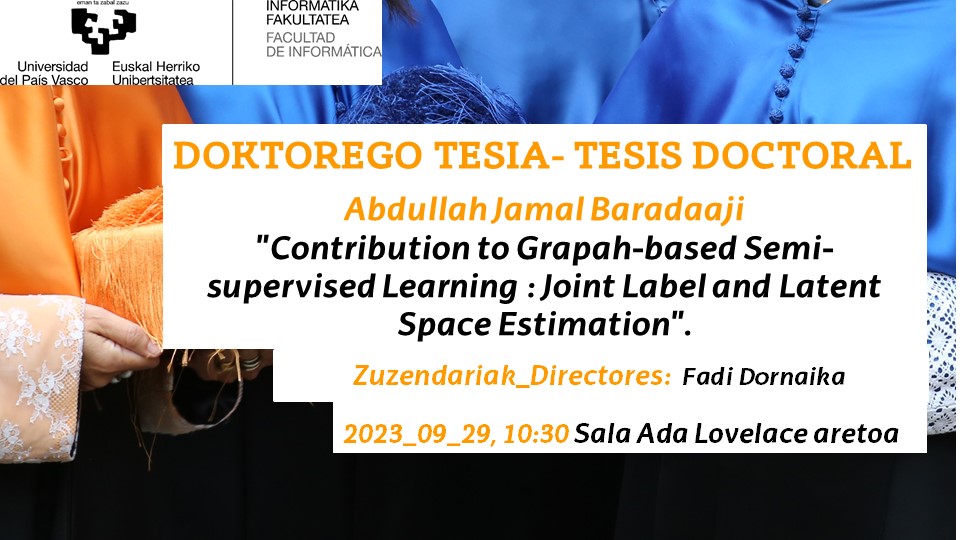
Abdullah Jamal Baradaaji: "Contribution to Grapah-based Semi-supervised Learning : Joint Label and Latent Space Estimation".
Zuzendariak_Directores: Fadi Dornaika.
2023_09_29, 10:30 Sala Ada Lovelace aretoa.
Abstract:
"Graph-based semi-supervised learning is a model from the field of machine learning that focuses on developing algorithms that allow computers to learn from data and make predictions or decisions. Datasets play a critical role in semi-supervised learning as they are used to train, evaluate, and validate models. These datasets contain both labeled and unlabeled data, and techniques such as graph-based methods and label propagation leverage both types of data. By leveraging the underlying graph structure, these methods aim to improve performance on learning tasks. Graph-based embedding approaches are effective in reducing dimensionality and extracting relevant features. Graphs provide valuable structural information at local and global levels. In scenarios where data lacks labels, graph-based semi-supervised learning (GBSSL) is particularly valuable.
This work focuses on investigating and proposing different methods for developing robust models in the field of graph-based semi-supervised learning. Throughout the research, we have explored various approaches and techniques to improve the performance and effectiveness of these models. By exploiting the inherent structure and relationships in graph data, we aimed to improve the accuracy and reliability of predictions.
The main contributions of the thesis are as follows.
{\bf {Firstly}}, this thesis presents a model for joint inference of soft labels and linear metric learning. This type of criterion, which simultaneously computes the class indicator matrix (soft labels) and the linear projection (latent space), would lead to better discriminative SSL models. The methods maximize the local margins within the projection space. In addition, both the estimated labels and the transformed data are subject to smoothing constraints. {\bf {Secondly}}, we introduce a new method for graph-based semi-supervised learning that estimates labels and linear transformations simultaneously. This is achieved by combining two types of graphs: the data graph based on the data samples and an additional label graph derived from the predicted soft labels. By using these hybrid and adaptive graphs, the inclusion of monitoring information is improved, resulting in an improved discriminative linear transformation. This model applies two types of regularization: one for the labels and one for the transformed data. We refer to this as automatic weighted graph fusion, which involves a weighted combination without explicit coefficients. The fusion coefficients are estimated automatically during the iterative optimization of the objective function. {\bf {Thirdly}}, we propose a unified semi-supervised model in which a low-rank data graph, the soft labels, and the latent subspace are jointly estimated with an objective function. The proposed system exploits the synergy between the graph structure and the data representation in terms of soft labels and latent features. This improves the monitoring information and leads to a better discriminative linear transformation.
We extensively tested and validated our hypotheses in several studies and extended our experiments and analyzes to demonstrate the robustness of our results. It is worth noting that our hypotheses outperformed many competing semi-supervised methods. Through extensive experiments and comparisons with other approaches on various benchmark datasets, we validated the superiority of our proposal over numerous state-of-the-art graph-based semi-supervised algorithms, including those that perform joint estimation of labels and latent space. In addition, we evaluated the performance of our model against other methods on large datasets to provide a comprehensive assessment of its capabilities.
Keywords: Graph-based embedding, discriminant embedding, soft labels, semi-supervised learning, pattern recognition, image categorization, auto-weighted graph fusion, structured data."